Shortcuts
Title Page
Breaking e-Banking CAPTCHAsShujun Li1, Syed Amier Haider Shah2, Muhammad Asad Usman Khan2, Syed Ali Khayam2, Ahmad-Reza Sadeghi3 and Roland Schmitz4
1 University of Konstanz, Germany
2 National University of Science and Technology (NUST), Pakistan
3 Ruhr-University of Bochum, Germany
4 Stuttgart Media University, Germany
© ACM (2010). This is the author’s version of the work. It is posted here by permission of ACM for your personal use. Not for redistribution. The definitive version has been published by ACM in Proceedings of 26th Annual Computer Security Applications Conference (ACSAC 2010, Austin, Texas, USA, December 6-10, 2010), pp. 171-180.
Abstract
Many financial institutions have deployed CAPTCHAs to protect their e-banking systems from automated attacks. In addition to traditional CAPTCHAs for login, CAPTCHAs are also used to prevent malicious manipulation of e-banking transactions by automated Man-in-the-Middle (MitM) attackers. Despite serious financial risks, security of e-banking CAPTCHAs is largely unexplored. In this paper, we report the first comprehensive study on e-banking CAPTCHAs deployed around the world. A new set of image processing and pattern recognition techniques is proposed to break all e-banking CAPTCHA schemes that we have found over the Internet, including three e-banking CAPTCHA schemes for transaction verification and 41 schemes for login. These broken e-banking CAPTCHA schemes are used by a large number of financial institutions worldwide, which are serving hundreds of millions of e-banking customers. The success rate of our proposed attacks are either equal to or close to 100%. We also discuss possible enhancements to these e-banking CAPTCHA schemes and show some essential difficulties of designing e-banking CAPTCHAs that are both secure and usable.Links
- BibTEX: http://www.hooklee.com/Papers/ACSAC2010.txt
- PDF Edition (Published Paper): http://www.hooklee.com/Papers/ACSAC2010.pdf (781KB)
- Presentation at ACSAC2010: http://www.hooklee.com/Papers/ACSAC2010-Slides.pdf (1.05MB)
- PDF Edition (Full Paper): http://www.hooklee.com/Papers/ACSAC2010_Full.pdf (1.29MB)
- HTML Edition (Full Paper): http://www.hooklee.com/Papers/Data/eBankingCAPTCHAs/Full-HTML/eBankingCAPTCHAs.html
e-Banking CAPTCHAs
CAPTCHAs have been widely deployed by many financial institutions around the world to protect their customers from automated online password attacks. Some financial institutions have also deployed CAPTCHAs for transaction verification, whose aim is to defeat automated Man-in-the-Middle (MitM) attack. As far as we know, there is no public report about deployment of different e-banking security measures in the world banking sector. To check how many financial institutions have deployed e-banking CAPTCHAs, we looked into the Web sites of many financial institutions around the world. The following is a summary of e-banking CAPTCHAs we found and analyzed in this research.- 30 major banks in China
- Hundreds of small and medium-sized banks in Germany
- Thousands of small and medium-sized banks and credit unions in USA
- Four credit unions in Australia
- A Swiss bank with branches in many other countries in Europe, North America, Asia and Africa
- A Pakistani bank
- A Central American private bank with customers in Europe, Asia, Australasia and Africa
Basic Tools Used for Our Attacks
The tools we used for developing our attacks are well-established image processing and pattern recognition techniques, mainly including the following:- k-means clustering: for segmenting objects from a CAPTCHA image
- Morphological image processing: for removing noises/decoy objects and refining shapes of segmentation
- (simplified) Hough transform: for detecting random grid lines used in some e-banking CAPTCHA schemes
- Digital image inpainting: for removing unwanted objects from a CAPTCHA image
- Character segmentation: for extracting characters from a CAPTCHA image
- Character recognition: for recognizing characters in a CAPTCHA image
- 2-D correlation
- CW-SSIM (a translation-invariant image quality assessment method, also robust against small rotation and scaling)
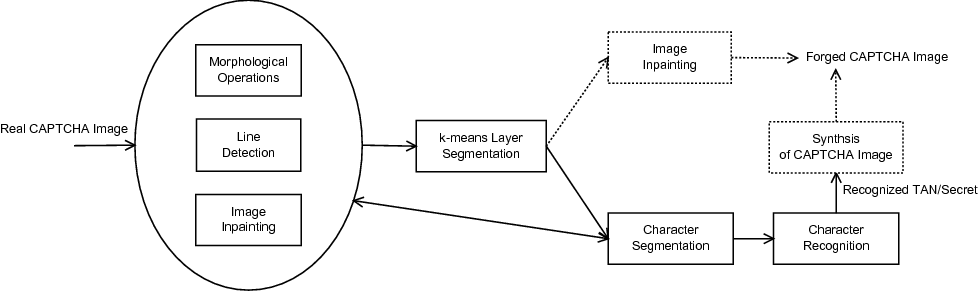
Our Results
All of our attacks were mainly implemented in MATLAB, a compiled programming language and an interactive programming environment. Part of the program was written in Java and C to overcome some functional limitations of MATLAB, which is then incorporated into our main code via MATLAB's external interfaces for Java and C.Breaking a German e-banking CAPTCHA Scheme
We developed two practical attacks on an e-banking CAPTCHA scheme used by around 800 German banks. The success rates of both attacks are 100% for 100 test images we collected from real bank accounts.Note: This e-banking CAPTCHA scheme has a specific name. To avoid exposing the name of the affected banks, we use "GeCaptcha" (German e-banking CAPTCHA) as the pseudonym of this scheme.
How does GeCaptcha work?
Before the user can make any online transactions, he/she will get a paper list of n TANs from the bank.- The user fills transaction data on the Web page and clicks a button to send the data to the e-banking server.
- The e-banking server generates a GeCaptcha image which embeds the user's birthday, the transaction data, a TAN index and the current time, and then sends it to the user.
- The user recognizes the TAN index, looks into her list of TANs, enters the TAN close to the TAN index on the Web page and sends it to the e-banking server.
- The e-banking server checks if the received TAN is correct, and accepts or rejects the transaction request.
- Line 1: "GeCaptcha control picture for transfer";
- Line 2: "Amount in EUR: 999,99 Bank code: 10203040 Account number: 12345678";
- Line 3: "Please enter the TAN next to the index 158".

Inpainting based atatck
The first attack is based on image inpainting, which remove the real transaction data and repalce them with fake ones. This attack can run in real time. The average running time is around 250ms. A forged image with this attack is shown as follows.
Recognition based atatck
The second attack is based on character recognition, which recognize the user's birthday first offline and then forge fake GeCaptcha images based on other known information (in an online mode). CW-SSIM is used to build the recognizer. The online attack part can run in real time, and the average running time is around 190ms. The offline attack part is relatively slow (around 5 seconds).The segmented birthday from the above GeCaptcha image is shown in the following. It can be correctly recognized as "14.10.1978".
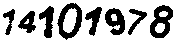
A forged image with this attack is shown as follows.

Breaking a Chinese e-banking CAPTCHA for transaction verification
A major Chinese bank has deployed a CAPTCHA scheme for transaction verification. This scheme is similar to but simpler than GeCaptcha.We developed one practical attack on this Chinese e-banking CAPTCHA scheme. We tested the attack on 100 test images we collected from a real bank account, and achieved a success rate of 100%. The attack can run in real time and the average running time is less than 150ms.
How does this CAPTCHA work?
- The user fills transaction data on the Web page and clicks a button to send the data to the e-banking server.
- The e-banking server generates a CAPTCHA image which embeds the receiver's account number and the receiver's name. Four digits in the receiver's name are randomly selected and highlighted in color and in bigger font size.
- The user recognizes the bigger color TAN digits, enters the TAN on the Web page and sends it to the e-banking server.
- The e-banking server checks if the received TAN is correct, and accepts or rejects the transaction request.
- Line 1: "receiver's account: 800167645271613670";
- Line 2: "receiver's name: 冯七";
- Line 3: "TAN Please input the big digits in red in the receiver's account".

Recognition attack
Since the TAN is part of the receiver's account, the image inpainting based attack on iTANplus does not work here. But the recognition based attack still works. Instead of CW-SSIM, 2-D correlation is used to build the recognizer.The following is the TAN digits segmented from the above CAPTCHA image, which can be easily recognized as "1670" by a coorelation-based method.
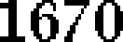
Breaking another Chinese e-banking CAPTCHA for transaction verification
Another major Chinese bank has also deployed a CAPTCHA scheme for transaction verification. This scheme is also similar to and simpler than iTANplus.The two attacks on GeCaptcha can both be generalized to break this Chinese CAPTCHA scheme. The generalized attacks can both achieve a success rate of 100% on 103 test images collected from real bank accounts.
How does this CAPTCHA work?
- The user fills transaction data on the Web page and clicks a button to send the data to the e-banking server.
- The e-banking server generates a CAPTCHA image which embeds the receiver's account number, the receiver's name, amount, and a 5-digit random TAN.
- The user recognizes the bigger color TAN digits, enters the TAN on the Web page and sends it to the e-banking server.
- The e-banking server checks if the received TAN is correct, and accepts or rejects the transaction request.
- Line 1: "Attention! Please check the following information carefully";
- Line 2: "receiver's account: 4402209009066183922";
- Line 3: "Receiver's name: 张三";
- Line 4: "Amount: 100.00".
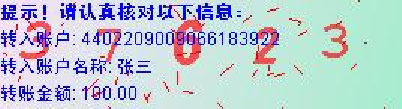
Inpainting based attack
This attack can run in real time and the average running time is around 200ms. The following is a forged image with this attack.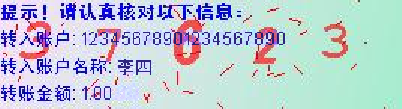
Recognition based attack
This attack is relatively slow, and the average running time is around 6-7 seconds. Note that this attack does not need to be in real time, because the CAPTCHA challenges are supposed to be solved by (slow) human users. The following is the segmented TAN from the above CAPTCHA image, which can be correctly recognized as "37623".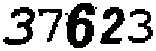
Breaking login CAPTCHAs
We have also developed segmentation attacks on all e-banking login CAPTCHA schemes we found. For each scheme, we tested each segmentation attack on at least 60 test images to confirm their robustness. The following is a table of all e-banking login CAPTCHA schemes we have analyzed.Financial institution(s)/e-banking login CAPTCHA scheme | CAPTCHA image(s) | Segmentation result(s) | Tool(s) used, Weakness(es) exploited | Success rate |
13 German banks | ![]() | ![]() | 3-means clustering, morphological operations | 60∕60 = 100% |
Hundreds other German banks | ![]() | ![]() | 2-means clustering, line detection, image inpainting | 60∕60 = 100% |
A Swiss bank with branches in Europe, Asia, North America and Africa (a Pakistani bank is also using the same system) | ![]() | ![]() | 2-means clustering | 60∕60 = 100% |
A bank based in Latin America with branches in Europe, Asia, Australasia and Africa | ![]() ![]() ![]() ![]() | ![]() ![]() ![]() ![]() | 2-means clustering | 63∕63 = 100% |
Old CAPTCHA scheme of the above bank | ![]() | ![]() | 2-means clustering | 60∕60 = 100% |
US e-banking CAPTCHA 1* | ![]() | ![]() | 2/3-means clustering, line detection, image inpainting | 209∕209 = 100% |
US e-banking CAPTCHA 2* | ![]() | ![]() | 4-means clustering, morphological operations | 71∕71 = 100% |
US e-banking CAPTCHA 3* | ![]() | ![]() | morphological operations, static color of foreground | 60∕60 = 100% |
US e-banking CAPTCHA 4* | ![]() | ![]() | 3-means clustering | 115∕115 = 100% |
US e-banking CAPTCHA 5* | ![]() | ![]() | 3-means clustering | 92∕92 = 100% |
US e-banking CAPTCHA 6* | ![]() | ![]() | 2-means clustering | 61∕61 = 100% |
US e-banking CAPTCHA 7* | ![]() | ![]() | 2/3-means clustering | 60∕60 = 100% |
A CU in Australia | ![]() | ![]() | 3-means clustering, morphological operations | 60∕60 = 100% |
Another Two CUs in Australia | ![]() | ![]() | 3-means clustering, morphological operations | 60∕61 ≈ 98.4% |
Yet another CU in Australia | ![]() | ![]() | 3-means clustering, morphological operations | 60∕60 = 100% |
Chinese e-banking CAPTCHA 1 | ![]() | ![]() | 3-means clustering | 60∕60 = 100% |
Chinese e-banking CAPTCHA 2 | ![]() | ![]() | 2-means clustering, image inpainting, static color of noises | 60∕60 = 100% |
Chinese e-banking CAPTCHA 3 | ![]() | ![]() | 4-means clustering, morphological opening | 62∕62 = 100% |
Chinese e-banking CAPTCHA 4 | ![]() | ![]() | morphological cleaning, character intensity < 120 | 61∕61 = 100% |
Chinese e-banking CAPTCHA 5 | ![]() | ![]() | 3-means clustering, morphological cleaning | 59∕60 ≈ 98.3% |
Chinese e-banking CAPTCHA 6 | ![]() | ![]() | static colors of foreground, background and noises | 61∕61 = 100% |
Chinese e-banking CAPTCHA 7 | ![]() | ![]() | grayscale foreground vs. colored noises | 60∕60 = 100% |
Chinese e-banking CAPTCHA 8 | ![]() | ![]() | 2-means clustering | 60∕60 = 100% |
Chinese e-banking CAPTCHA 9 | ![]() | ![]() | 2-means clustering, morphological opening | 61∕61 = 100% |
Chinese e-banking CAPTCHA 10 | ![]() | ![]() | 2-means clustering | 60∕60 = 100% |
Chinese e-banking CAPTCHA 11 | ![]() | ![]() | 2-means clustering | 64∕64 = 100% |
Chinese e-banking CAPTCHA 12 | ![]() | ![]() | segmentation labels available as color indices | 64∕64 = 100% |
Chinese e-banking CAPTCHA 13 | ![]() | ![]() | 3-means clustering | 60∕60 = 100% |
Chinese e-banking CAPTCHA 14 | ![]() | ![]() | morphological operation, foreground intensity always darker than 128 | 60∕60 = 100% |
Chinese e-banking CAPTCHA 15 | ![]() | ![]() | 2-means clustering | 110∕110 = 100% |
Chinese e-banking CAPTCHA 16 | ![]() | ![]() | 2/3-means clustering | 60∕60 = 100% |
Chinese e-banking CAPTCHA 17 | ![]() | ![]() | 2-means clustering | 62∕62 = 100% |
Chinese e-banking CAPTCHA 18 | ![]() | ![]() | 2-means clustering, morphological operations | 58∕61 ≈ 95.1% |
Chinese e-banking CAPTCHA 19 (deployed by two banks) | ![]() | ![]() | 3-means clustering, morphological filling | 63∕63 = 100% |
Chinese e-banking CAPTCHA 20 | ![]() | ![]() | 2-means clustering | 60∕60 = 100% |
Chinese e-banking CAPTCHA 21 (deployed by four banks) | ![]() | ![]() | 3-means clustering | 61∕61 = 100% |
Chinese e-banking CAPTCHA 22 | ![]() | ![]() | 2-means clustering | 60∕60 = 100% |
Chinese e-banking CAPTCHA 23 | ![]() | ![]() | 2-means clustering | 60∕60 = 100% |
Chinese e-banking CAPTCHA 24 | ![]() | ![]() | static character color (white) | 60∕60 = 100% |
Chinese e-banking CAPTCHA 25 | ![]() | ![]() | static character color (black) | 60∕60 = 100% |
Chinese e-banking CAPTCHA 26 | ![]() | ![]() | clearly separable background and foreground | 60∕60 = 100% |